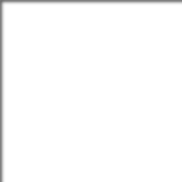
Holes in Some of Finance’s Critical Assumptions: A Dialogue with Massif Partners’ Kevin Harney (Part One)
Posted by Jason Apollo Voss on May 29, 2012 in Blog | 0 commentsIn finance even basic assumptions — like which technique to use to calculate prices for such financial instruments as derivatives or how you calculate rates of return for assets — determine how you see the world. If the techniques have flaws, then your understanding and insight into things as fundamental as prices and return are also flawed.
Chief among the flaws are Brownian motion used as a proxy of random movement, its use in calculating compound annual growth rates, and the valuation sensitivity of derivative instruments to volatility.
Serendipitously, several weeks ago for the first time, I met Kevin Harney — a true quant — and we both discovered that we have mutual interest in the holes in some of finance’s critical assumptions.
Kevin graduated with a master’s degree in mathematical finance from the University of Alberta in 1997. Furthermore, he has two degrees in mathematics and applied mathematics under his belt from University College, Galway, in Ireland. Not to mention his career experience, which ranges from managing the Interest Rate Derivative Pricing Technology group at JPMorgan to being the head of Asset Liability Management at JPMorgan’s Pension Advisory Group before moving to a similar role at Massif Partners, a quantitatively driven hedge fund. In other words, Kevin knows what he is talking about from the technical side, in terms of implementation of finance’s basic assumptions and their impact on results, as well as their use as investments and hedges.
Jason Voss: First, let’s talk about Brownian motion. Brownian motion is used in finance to calculate compound annual growth rates and to model them as well. In fact, one of the very first lessons of any finance course is: “This is how you calculate rates of return.” We all recognize its formula:
But most of us accept the technique without knowing that this is Brownian motion, questioning its assumptions, or understanding the origins of the technique.
Kevin Harney: Brownian motion originally referred to apparently random movements of particles at a microscopic level — this was before any decent models of atoms or molecules existed (which was not that long ago — we are talking early 19th century). The motion was driven by random molecular collisions — effectively heat/kinetic energy. As with many physical phenomena involving large numbers of components (consider the number of atoms in a single drop of water), there is a legitimate physical reason for converging to some type of predictable, random behavior or “distribution.”
What is the effect on financial models of using Brownian motion?
Without being trite, it makes the models tractable. Brownian motion as a stochastic process [i.e., random] is the B or W term seen in every stochastic differential equation [i.e., an equation designed to model change] — W being a Wiener process, which is a particular type of Brownian motion.
The Wiener process is the portion of the CAGR formula that indicates over how many time periods random events have occurred.
Brownian motion also assumes that random events, such as financial asset prices, unfold in a continuous fashion, when in fact they do not. If, for example, you are performing a discounted cash flow analysis and you assume that growth for the business is continuous — when in fact, it never is — then the modeled price is assured of being off. For example:
In the first line of the above chart, you can see the actual annual return percentage over the course of five years for a financial asset. In line two, you can see the actual value of that financial asset at the end of each year. Note that the returns are up, up, down, up, and then down again. This return pattern results in a compound annual growth rate of 5.51%. This calculation, however, assumes that the return pattern is smooth, but in fact it is not.
If you look at line three, it shows what the implied value of the asset is if you assume a CAGR of 5.51%. Last, line four shows the valuation error that you would make each year by using CAGR, or Brownian motion in a valuation model.
This, in a nutshell, is the problem with Brownian motion used as a model for returns, and any model that makes use of projected returns is going to be precisely wrong in its estimates.
Work by [Kiyoshi] Itō on stochastic calculus in the 1950s and his lemma set the groundwork for much of what was to come in terms of becoming facile with these stochastic processes and allowing their eventual widespread use. Of course, like all branches of mathematics, there are hundreds of years of thought which went into their development — from Pascal, through Einstein, Itō and then to Black/Scholes/Merton.
All it took then was access to computing power to ignite this particular stick of dynamite in the business world. It turns out that there are option-like features almost everywhere you look in any contract or obligation. And if there is something that looks like an option, then someone has modeled it as such and can tell you exactly how much it’s supposed to be worth.
What is the effect on derivative valuations by using models that are so sensitive to changes in volatility (e.g., delta and gamma)?
I don’t think sensitivity is the issue — there are parameters that go into any of these stochastic equations and the solutions are all continuous and nicely behaved. The issue is the set of assumptions underlying the valuation model in the first place and whether they are appropriate: in particular, the assumption about normality that is embedded in standard Brownian motion, and the fact that volatility of the underlying asset is supposed to be constant. Almost from the outset, there was the recognition that volatility is not constant in practice, and then we’ve spent decades building very complex volatility models to compensate for this mismatch between theory and reality — when the lack of normality is a large contributor to that divergence. But taking away normality makes it a very difficult problem to solve.
What are among the difficulties?
Normality gives you closed form solutions for many pricing problems. However advances in computational speed and parallel processing allow you to use more complex non-closed form solutions.
What are some of the other factors in derivative valuations upset by the assumption of normality?
Of course, there are plenty of other issues — such as the Black-Scholes assumptions around continuous cost-free hedging of a replicating portfolio. Don’t get me wrong — these models are beautiful in their abstraction. The flaw has been in taking a wonderful branch of mathematics (though the physicists will claim it’s theirs, and then the economists will say the same) and applying it to a set of processes where there are human actors with very unpredictable behavior and significant agency issues (mortgage underwriters, CDS and CDO issuers, etc.). The problem has not been derivative pricing models, per se, but the unerring belief in our ability to capture the behavior of market actors (or assume that they are all rational, well-intentioned, etc.).
In tandem, the advent of powerful parallel processing and data mining techniques, has led us on the quest for more analysis — more sensitivities, more risk factors — rather than an honest assessment of the legitimacy and limitations of what’s being calculated.
At the end of the day, for “vanilla” derivatives, where there’s a liquid market, the price is known and bid-offers are very tight, and the implied volatility is a convenient way of expressing the price. The market sets the price and effectively calibrates the model to allow for off-market pricing.
An “exotic” derivative is, by definition, one where there is no liquid market. And dealers put aside larger reserves to deal with any potential mispricing issues that may result. Of course, through time, what was exotic becomes, by definition, vanilla. But just because there’s a liquid market, it doesn’t make risks go away. It just means there’s general agreement on the methodology and assumptions used in pricing. Whether it holds up or not in practice remains a separate issue. I think it’s fair to say that the CDS market was considered quite vanilla until defaults soared. “Vanilla,” like beauty, is in the eye of the beholder.
In part two of this interview Kevin Harney, we will discuss further the effects of these choices and what can be done differently.
Originally published on CFA Institute’s Enterprising Investor.