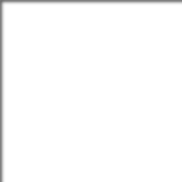
Skills That Separate You as an Investment Manager: Discernment
Posted by Jason Apollo Voss on Oct 7, 2014 in Best of the Blog, Blog, Skills That Separate You as an Investment Manager | 0 commentsPrevious posts in this series have included important, fairly high-level skills that separate you as an investment manager — such as introspection, creativity, intuition, and decisiveness. But what about a skill that lets you roll up your sleeves and get your hands dirty? Discernment is such a skill.
Many investment managers and research analysts think that one of their responsibilities is to discover the very best mental model for their work. For instance, much academic research, even in CFA Institute’s archives, is focused on identifying which valuation model is the best predictor of future performance. I say: the best tool for the job is a better philosophy. Skilled investment managers not only know about and understand many different mental models, they also know when to deploy them — this is discernment. I consider discernment to be the combination of knowledge, memory, creativity, intuition, non-attachment, and awareness.
Because there is so much research focused on the use of valuation models, let me instead share with you several mental models that analysts and portfolio managers deploy unconsciously and that subsequently interfere with discernment.
Mechanical Models
Mechanical models are those structured as long chains of logical effects flowing from causes. Typical of this kind of model is the discounted cash-flow valuation model that projects a flat revenue growth rate, like 7%, each year for some time horizon, say five years. These models are qualitative, too. One such mental model is too much money supply = inflation.
Mental models of this kind are the natural outgrowth of a scientific philosophy — rational determinism — that dominated the more physical sciences, like physics, chemistry, and biology, for centuries. If you learned mathematics or science in secondary school, this is most likely the way you were taught to view the world. Unfortunately, investing is a complex merging of soft — not hard — sciences with probability theory, mathematics, economics, philosophy, intuition, and personal choice.
These models are fine and they have their place, but they have a fatal flaw: they are mechanistic. This is a flaw because machines are closed-state phenomena. I build a machine for a specific task to be deployed in a limited number of circumstances. Machines have a limited number of parts and are designed to work together within a confined housing with limited interaction with non-state specific factors. Highly sophisticated watches are not meant to choose the next CEO of a business.
Despite the fact that mechanical models miss almost every state change, mechanical thinking predominates the thinking of most investment managers. As an aside, I believe that most managers see robotic, emotionless, cold logic as the ideal model. If you read a research report and see a long logic chain where the first cause leads inevitably to an effect many, many steps removed from the initial event, you are likely seeing an analyst in robot mode. They may be correct, but life is rarely so neat and prescriptive.
So when is the best time to deploy mechanical models? When a system has few outside influences, the outcome of interactions between different parts of the system is highly predictable, and requires few state changes. In other words, these models are excellent for analyzing facts. This may seem odd given the preceding dialogue, but facts, by definition, are closed-state. Put another way, facts by definition are things that occurred in the past. There is no such thing as a future fact. Thus, do use financial ratios — mechanical models — to analyze past business performance.
Believe it or not, mechanical models can be very good state change predictors when that is the only thing they are asked to predict. One such example is that raising prices likely leads to lower demand. Note, however, the mechanical model is probably not going to be a good predictor of the relative level of demand change.
Evolutionary Models
Evolutionary models recognize that the state of a system is ever-changing; changing toward a singular point in the future. The singular point of evolutionary models is victory at the end of the time horizon under consideration. This victory has many definitions, but one is the lack of predation: we won!
In investing, many look for businesses that smite the competition by constantly evolving. EPS at worst has a second derivative that is positive, and at best has a third derivative that is positive, too. In other words, it is always up, up, and away in evolutionary models! There may be some fluctuation in the growth rate, but evolution marches on.
Evolutionary models miss the mark because they overwhelmingly assume the present state is better than the past state. That is, they always assume the present state is the result of an evolution from a previous, not-as-evolved state. So ancient people and their cultures must necessarily be primitive. Why? Because they came before our culture. Yet the evolutionary model cannot make sense of Europe’s Dark Ages, which are believed to be a period not as evolved as that of Ancient Greece or Rome. In finance, we knew 2007 must be better than 1997 because of evolution and because profit growth told us it was better. Glass-Steagall had to give way to evolutionary forces. Such a fixed evolutionary mindset must have been devastated by the devolution that was the Great Recession.
Evolutionary models are effective at describing new systems that are in flux: where there is struggle and a desire to end or limit struggle. In finance, evolution serves as the legitimate model for valuing a business, so long as it is tempered with the understanding that devolution is also possible. In other words, that dominant company will eventually miss an earnings estimate.
Adaptive Models
Complementing evolutionary models — and in stark contrast to mechanical models — is adaptation. Here investment managers recognize that initial causes do not usually lead inexorably to certain effects as predicted by mechanical thought, or even to improvements. Instead, adaptive models predict response to initial stimuli. Further, these models accept that adaptive responses frequently dampen the expected effects of initial causes. So yes, quantitative easing puts more money in the global economy, but it does not necessarily have to lead to inflation as participants in the system may adapt to the new reality.
When adaptive models are combined with either evolutionary or mechanical models, more realistic predictions are frequently made. However, these models can make errant predictions because anticipating the actual nature of adaptive responses adds layers of complexity to modeling.
Ecosystem Models
Ecosystem models describe the complex inner workings of large systems by combining mechanical, evolutionary, and adaptive models into a whole. GDP models are an example of ecosystem models.
Ecosystem models are not sensitive to the errors of mechanical models in which getting the initial assumption incorrect means your entire model is wrong. Ecosystem models are robust enough to blur away the damage from such an error. These models also do not have the same problem that evolutionary models do in not accounting for the destruction of players in the system.
Unfortunately, ecosystem models often lack specificity (the hallmark of mechanical models) because they are too complex to lead to understanding. Also, if the interrelationships are not well understood, they lead to runaway outcomes, whether too much success or too much destruction.
How to Be More Discerning
Spend some time in quiet introspection to begin to log how you think. All of us have preferred time- and battle-tested mental models. Begin to notice which models are your defaults. I am guessing that one of the models above is a part of your early firing mental apparatus. Before using these models blindly, ask yourself, “Is this an appropriate use of my model?” This is discernment.
Photo credit: Stephen Campbell, 2014
Originally published on CFA Institute’s Enterprising Investor.