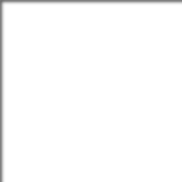
Behavioral Finance – Bias Deep Dive: Representativeness
Posted by Jason Apollo Voss on Sep 15, 2020 in Behavioral Bias Deep Dive, Blog | 0 commentsWe are now in the home stretch of my series of deep dive articles on behavioral finance. Up this week is representativeness bias. One complaint I have with some of behavioral finance is their changing of terms we all know and giving them new titles. This is especially true of representativeness bias which is essentially the use of stereotypes when judging investment phenomena.
In several weeks I conclude the deep dive by landing with A Theory of Behavioral Finance.
A Helpful Mnemonic Device: LOCHAARM
To my mind the major behavioral biases are:
- Loss aversion
- Overconfidence
- Confirmation
- Herding
- Anchoring
- Availability
- Representativeness
- Mental accounting
The above mnemonic device may prove helpful for remembering the major behavioral biases. Specifically, the above is LOC HAARM, brain lock that harms investment performance.
Representativeness Bias: Origins
Like much of behavioral economics, representativeness bias was first described by that dynamic duo, Tversky and Kahneman.[1] Specifically they state:
“We submit that people view a sample randomly drawn from a population as highly representative, that is, similar to the population in all essential characteristics. Consequently, they expect any two samples drawn from a particular population to be more similar to one another and to the population than sampling theory predicts, at least for small samples.”
Said in more everyday language, representativeness is very similar to making judgments based on stereotypes, prototypes, and archetypes. It may be that a Tory politician is not the best for the economy. Or that a Democrat may be tougher on crime than a Republican.
Representativeness appears in many places, particularly in sports where contracts are negotiated based on last year’s performance, as well as expectations for teams and their record of winning.
We make many judgments based on representativeness. We cast our presidential vote for the person who looks presidential; people who are attractive are judged to be more intelligent; people who are good speakers are judged to be smarter; and CEOs tend to be taller than average and with deeper voices. These are just a few of the manifestations of representativeness.
Representativeness Bias: Nuances
Again, the realizations of representativeness bias about preferences and prejudices being detrimental to understanding has been well known throughout human history. Nonetheless, researchers have extended understanding with important points. For example, a critical point to understand about representativeness bias is that just because something resembles expectations about a population does not necessarily make it more likely.
More formally Kahneman and Tversky note that representativeness is especially likely in several specific situations:
- What is the probability that object A belongs to class B?
- What is the probability that event A originates from process B?
- What is the probability that process B will generate event A?[2]
Tversky and Kahneman provide an explicit example: “Steve is very shy and withdrawn, invariably helpful, but with little interest in people, or in the world of reality. A meek and tidy soul, he has a need for order and structure, and a passion for detail.”[3]
In this kind of study, they then go on to ask participants the likelihood that Steve has a particular profession, such as farmer, salesman, airline pilot, librarian, or physician. If you understand representativeness, then you likely guessed that most research subjects state that Steve is a librarian.
Representativeness Bias: Manifestations
Above, the example of Steve, the likely librarian has an expression in investing where job candidates are frequently hired because it is believed they will be a good “cultural fit.” That fit is assessed based on a résumé and several interviews. Interviewers go out of their way to invoke mental filters in search of indications that someone likely conforms to expectations.
The problem is that hiring the same kind of person over and over again means that you get the same results over and over again. Great, so long as investment performance is outstanding. But the same kind of thinking does not maximize the benefits of cognitive diversity over the long run. This is true, too, of hiring people from the same short list of universities where candidates are educated by the same suite of professors with the same suite of ideas.
Another manifestation of representativeness bias is how often in investing have we heard “Past performance is no indication of future performance?” Every prospectus and investment performance report is required to include such a disclaimer. In fact, numerous academic studies confirm that past performance is not predictive of future investment manager performance and if anything, it is a negative predicator, with poor past performance predicting superior future performance.
So, with all the warnings and the evidence against using past performance in selecting managers, what does everyone do? Use past performance! The emotional attachment to past performance overwhelms the compelling evidence of its lack of predictive power.
This is a classic example of representativeness bias. We make judgments using our internal image of what the person or situation should look like. In the above case, since the manager has performed well in the past, we easily jump to the conclusion that performance will be good in the future. That is, past performance is representative of future performance, even though it is not predictive.
Many investment managers base their expectations of future performance of securities and the businesses that issue them on the recent performance. In fact, financial statement analysis frequently serves as the basis for valuation models. If the valuation model is just an extrapolation of past performance then it is more vulnerable to representativeness bias than one that intentionally adjusts assumptions to test the actual performance of the business.
In investing the idea that a good company is a precursor to picking a good stock. That is, if the company is well run, with quality management, carries little debt, is innovative, and has grown large, it must be a good stock to buy. The qualities of the company are representative of the investment qualities of the stock. On the other hand, a weak company with lots of debt, teetering on bankruptcy, weak management, and falling sales must be a bad investment. If we like the company’s products, we should invest in its stock.
Note: this may sound like I am advocating abandoning investment philosophies. I am not. What I am saying is that to the degree that an investment philosophy distorts rather than reveals reality, it is biased. So, apply those investment philosophies in an unbiased way! And be willing to update the philosophy because reality is constantly in flux, and ideas, institutions, and people are adapting constantly, too.
Last, investors are frequently called upon to make snap judgments of consequence, and without much information. This is another example of how most behavioral biases can be summarized by a single realization: when data or logical reasoning can answer a question, people frequently do not make use of either.
Conclusion
Representativeness bias is among the easier biases to understand. It essentially says we actively pursue the creation of stereotypes in the form of our philosophy of investing, and then we also actively look for places to deploy it. Another version is judgment based on shallow assumptions, rather than data. Like the other biases discussed so far, representativeness bias provides conscious investors with alpha opportunity. For example, it is my opinion that unconstrained funds are likely to be less hampered by representativeness because the investment universe is larger and the only job is to make money. Whereas, the (dreaded) style box is likely to inject representativeness bias into investment decision making. Caveat emptor!
[1] Tversky, Amos, and Daniel Kahneman. “Belief in the Law of Small Numbers.” Psychological Bulletin, Vol. 76, No. 2 (1971): 105-110
[2] Tversky, Amos, and Daniel Kahneman. “Judgment under Uncertainty: Heuristics and Biases.” Science, New Series, Vol. 185, No. 4157 (1972): p. 1124
[3] Ibid. p. 1124